# Install TF-DF !pip install tensorflow tensorflow_decision_forests # Load TF-DF import tensorflow_decision_forests as tfdf import pandas as pd # Load a dataset in a Pandas dataframe. train_df = pd.read_csv("project/train.csv") test_df = pd.read_csv("project/test.csv") # Convert the dataset into a TensorFlow dataset. train_ds = tfdf.keras.pd_dataframe_to_tf_dataset(train_df, label="my_label") test_ds = tfdf.keras.pd_dataframe_to_tf_dataset(test_df, label="my_label") # Train a Random Forest model. model = tfdf.keras.RandomForestModel() model.fit(train_ds) # Summary of the model structure. model.summary() # Compute model accuracy. model.compile(metrics=["accuracy"]) model.evaluate(test_ds, return_dict=True) # Export the model to a SavedModel. model.save("project/model")
# Install YDF !pip install ydf -U import ydf import pandas as pd # Load a dataset with Pandas ds_path = "https://raw.githubusercontent.com/google/yggdrasil-decision-forests/main/yggdrasil_decision_forests/test_data/dataset/" train_ds = pd.read_csv(ds_path + "adult_train.csv") test_ds = pd.read_csv(ds_path + "adult_test.csv") # Train a Gradient Boosted Trees model model = ydf.GradientBoostedTreesLearner(label="income").train(train_ds) # Look at a model (input features, training logs, structure, etc.) model.describe() # Evaluate a model (e.g. roc, accuracy, confusion matrix, confidence intervals) model.evaluate(test_ds) # Generate predictions model.predict(test_ds) # Analyse a model (e.g. partial dependence plot, variable importance) model.analyze(test_ds) # Benchmark the inference speed of a model model.benchmark(test_ds) # Save the model model.save("/tmp/my_model") # Export the model as a TensorFlow Saved Model model.to_tensorflow_saved_model("/tmp/my_saved_model")
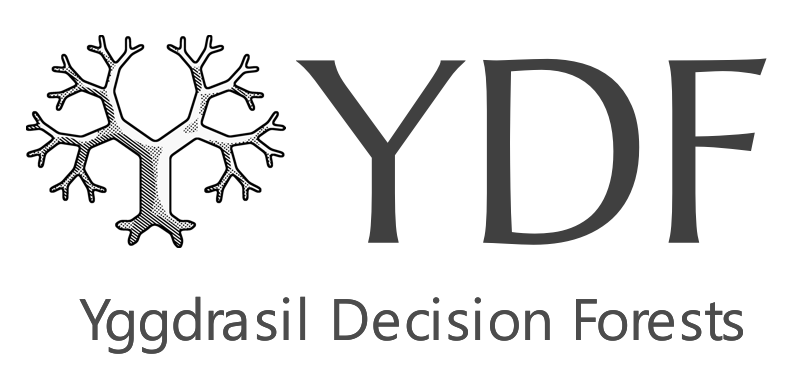
YDF is Google's new library to train Decision Forests.
YDF extends the power of TF-DF, offering new features, a simplified API, faster training times, updated documentation, and enhanced compatibility with popular ML libraries.
TensorFlow Decision Forests (TF-DF) is a library to train, run and interpret decision forest models (e.g., Random Forests, Gradient Boosted Trees) in TensorFlow. TF-DF supports classification, regression, ranking and uplifting.
Keywords: Decision Forests, TensorFlow, Random Forest, Gradient Boosted Trees, CART, model interpretation.Documentation & Resources
The following resources are available:
- Guides and tutorials
- API reference
- YDF documentation (also applicable to TF-DF)
- Google Developers' Decision Forests online class
Community