TFX is an end-to-end platform for deploying production ML pipelines
When you're ready to move your models from research to production, use TFX to create and manage a production pipeline.
How it works
A TFX pipeline is a sequence of components that implement an ML pipeline which is specifically designed for scalable, high-performance machine learning tasks. Components are built using TFX libraries which can also be used individually.
How companies are using TFX
Solutions to common problems
Explore step-by-step tutorials to help you with your projects.
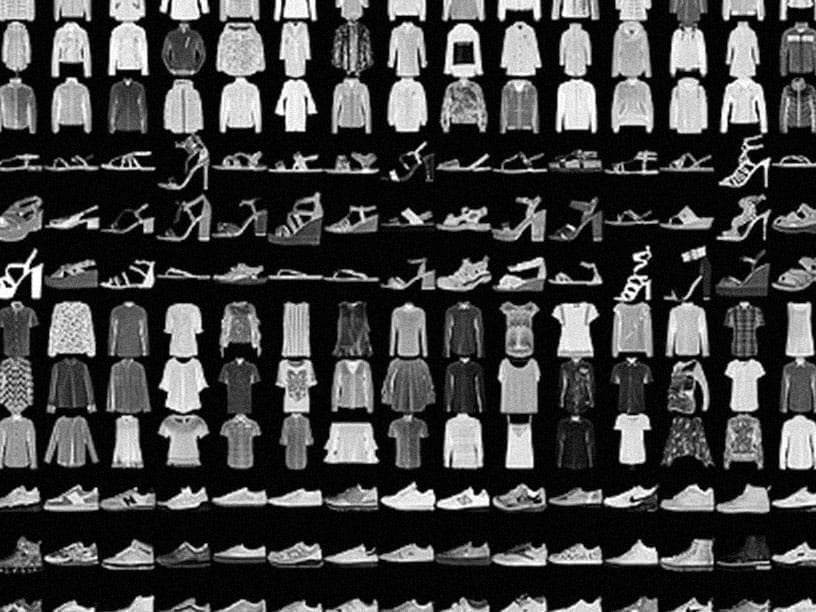
This guide trains a neural network model to classify images of clothing, like sneakers and shirts, saves the trained model, and then serves it with TensorFlow Serving. The focus is on TensorFlow Serving, rather than the modeling and training in TensorFlow.
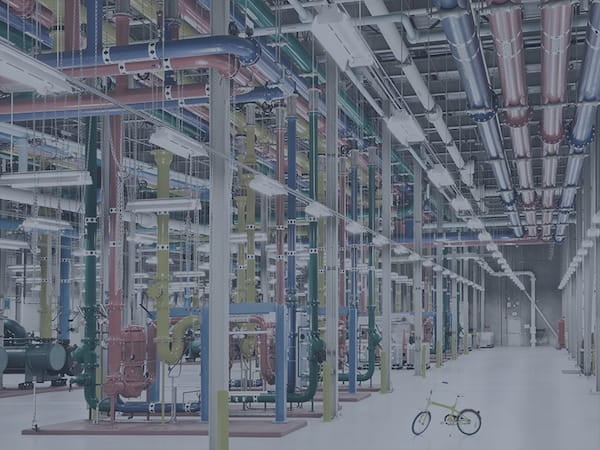
An introduction to TFX and Cloud AI Platform Pipelines to create your own machine learning pipelines on Google Cloud. Follow a typical ML development process, starting by examining the dataset, and ending up with a complete working pipeline.
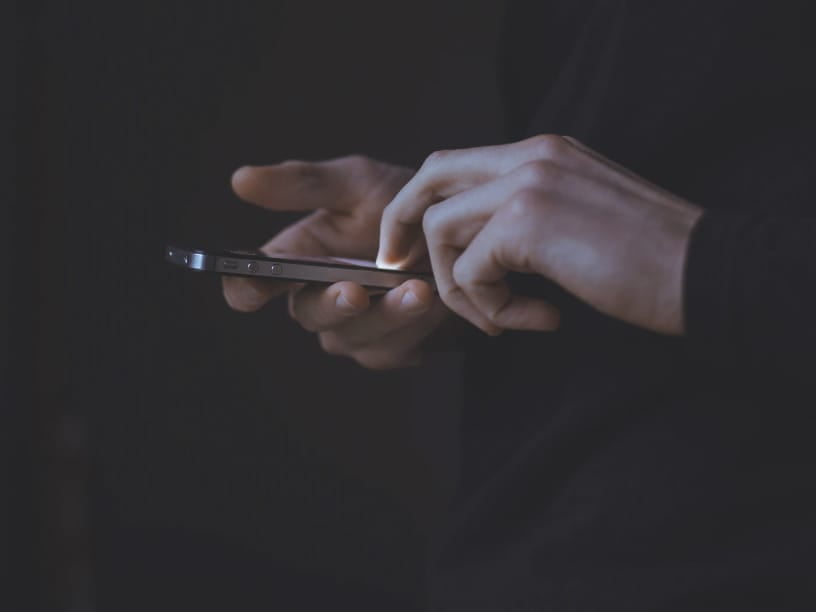
Learn how TFX can create and evaluate machine learning models that will be deployed on-device. TFX now provides native support for TFLite, which makes it possible to perform highly efficient inference on mobile devices.
News & announcements
Check out our blog and YouTube playlist for additional TFX content,
and subscribe to our TensorFlow newsletter to get the
latest announcements sent directly to your inbox.