Master your path
To become an expert in machine learning, you first need a strong foundation in four learning areas: coding, math, ML theory, and how to build your own ML project from start to finish.
Begin with TensorFlow's curated curriculums to improve these four skills, or choose your own learning path by exploring our resource library below.
The four areas of machine learning education
When beginning your educational path, it's important to first understand how to learn ML. We've broken the learning process into four areas of knowledge, with each area providing a foundational piece of the ML puzzle. To help you on your path, we've identified books, videos, and online courses that will uplevel your abilities, and prepare you to use ML for your projects. Start with our guided curriculums designed to increase your knowledge, or choose your own path by exploring our resource library.
TensorFlow curriculums
Start learning with one of our guided curriculums containing recommended courses, books, and videos.
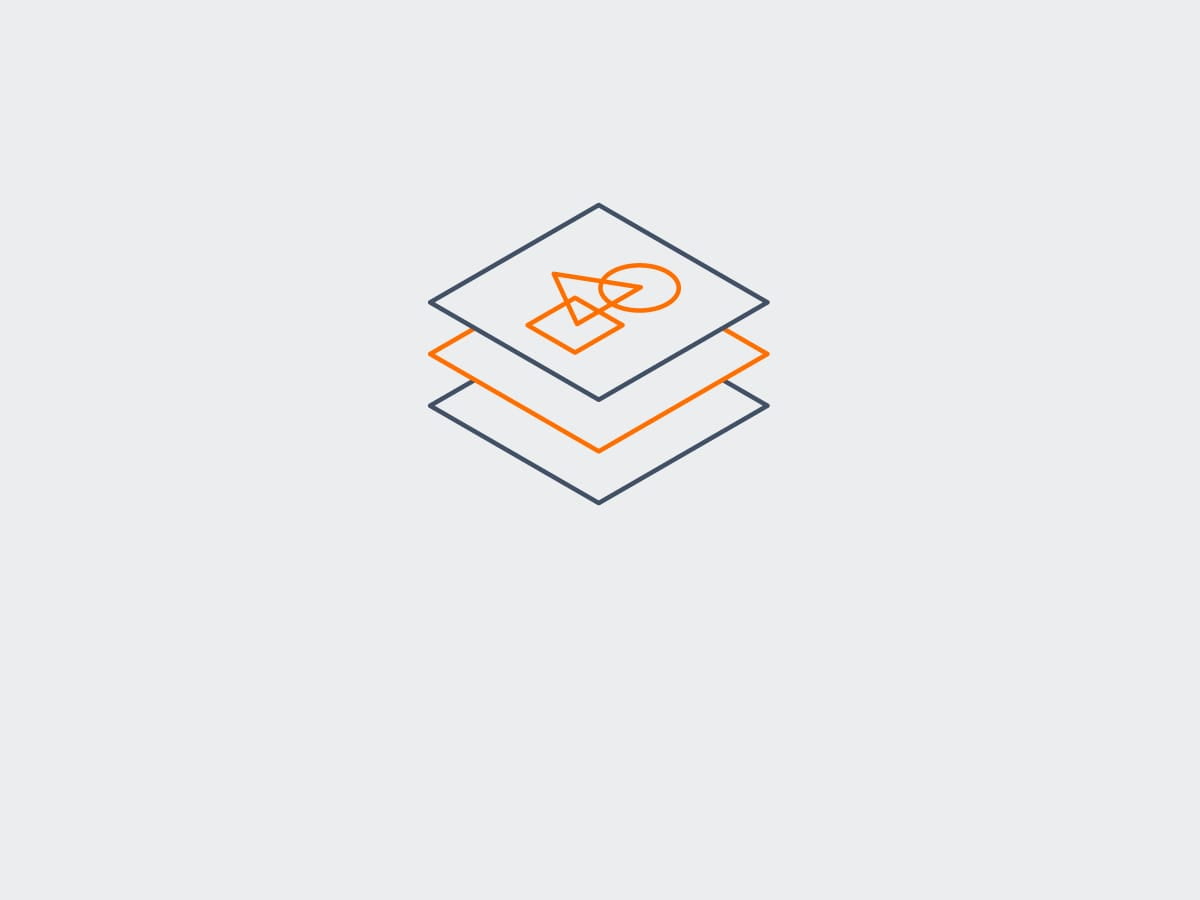
Learn the basics of ML with this collection of books and online courses. You will be introduced to ML and guided through deep learning using TensorFlow 2.0. Then you will have the opportunity to practice what you learn with beginner tutorials.
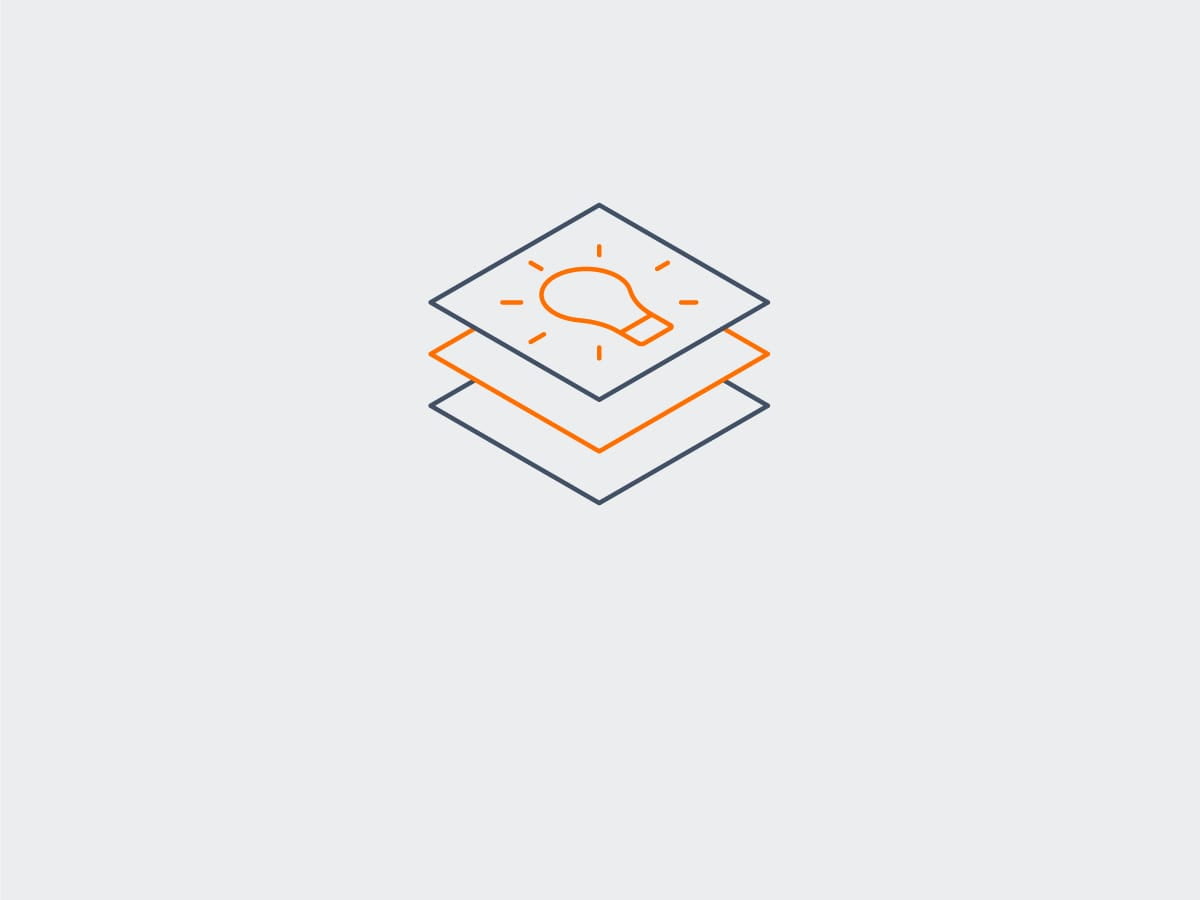
Once you understand the basics of machine learning, take your abilities to the next level by diving into theoretical understanding of neural networks, deep learning, and improving your knowledge of the underlying math concepts.
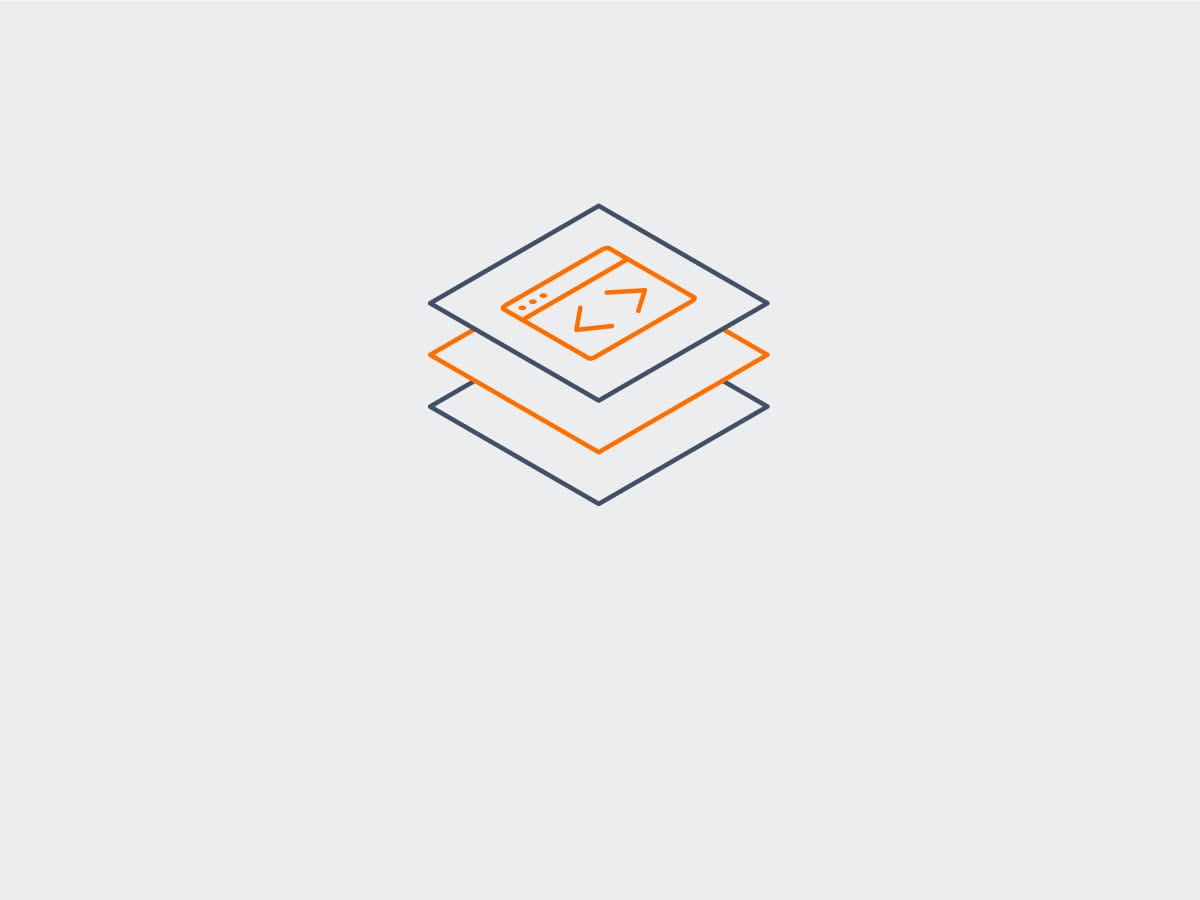
Learn the basics of developing machine learning models in JavaScript, and how to deploy directly in the browser. You will get a high-level introduction on deep learning and on how to get started with TensorFlow.js through hands-on exercises.
Educational resources
Choose your own learning path, and explore books, courses, videos, and exercises recommended by the TensorFlow team to teach you the foundations of ML.
Books
Reading is one of the best ways to understand the foundations of ML and deep learning. Books can give you the theoretical understanding necessary to help you learn new concepts more quickly in the future.
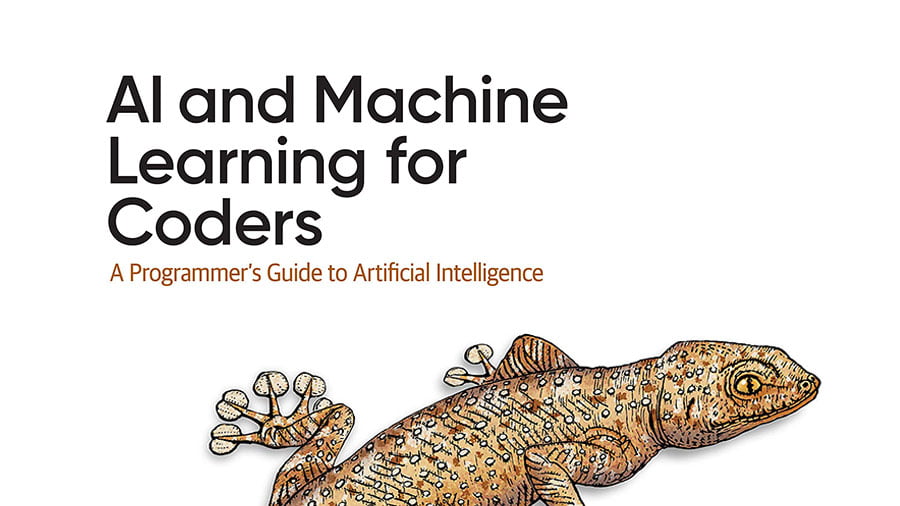
This introductory book provides a code-first approach to learn how to implement the most common ML scenarios, such as computer vision, natural language processing (NLP), and sequence modeling for web, mobile, cloud, and embedded runtimes.
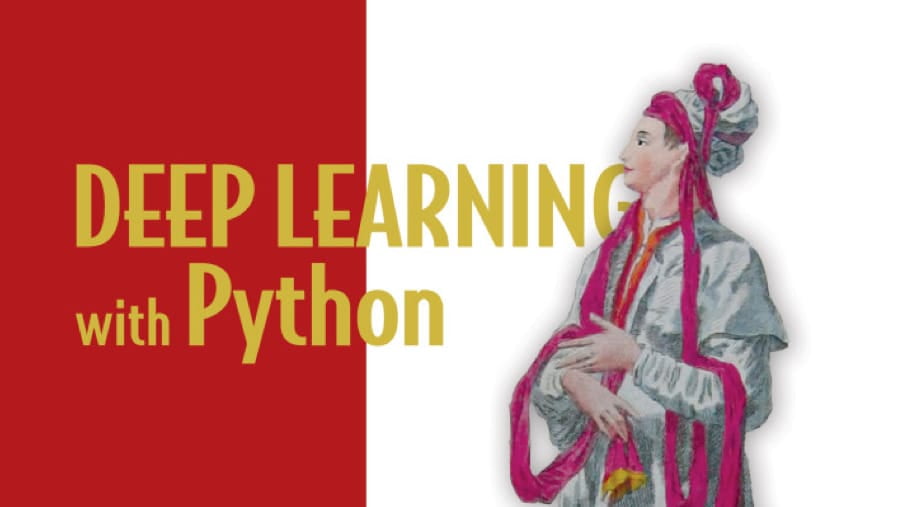
This book is a practical, hands-on introduction to Deep Learning with Keras.
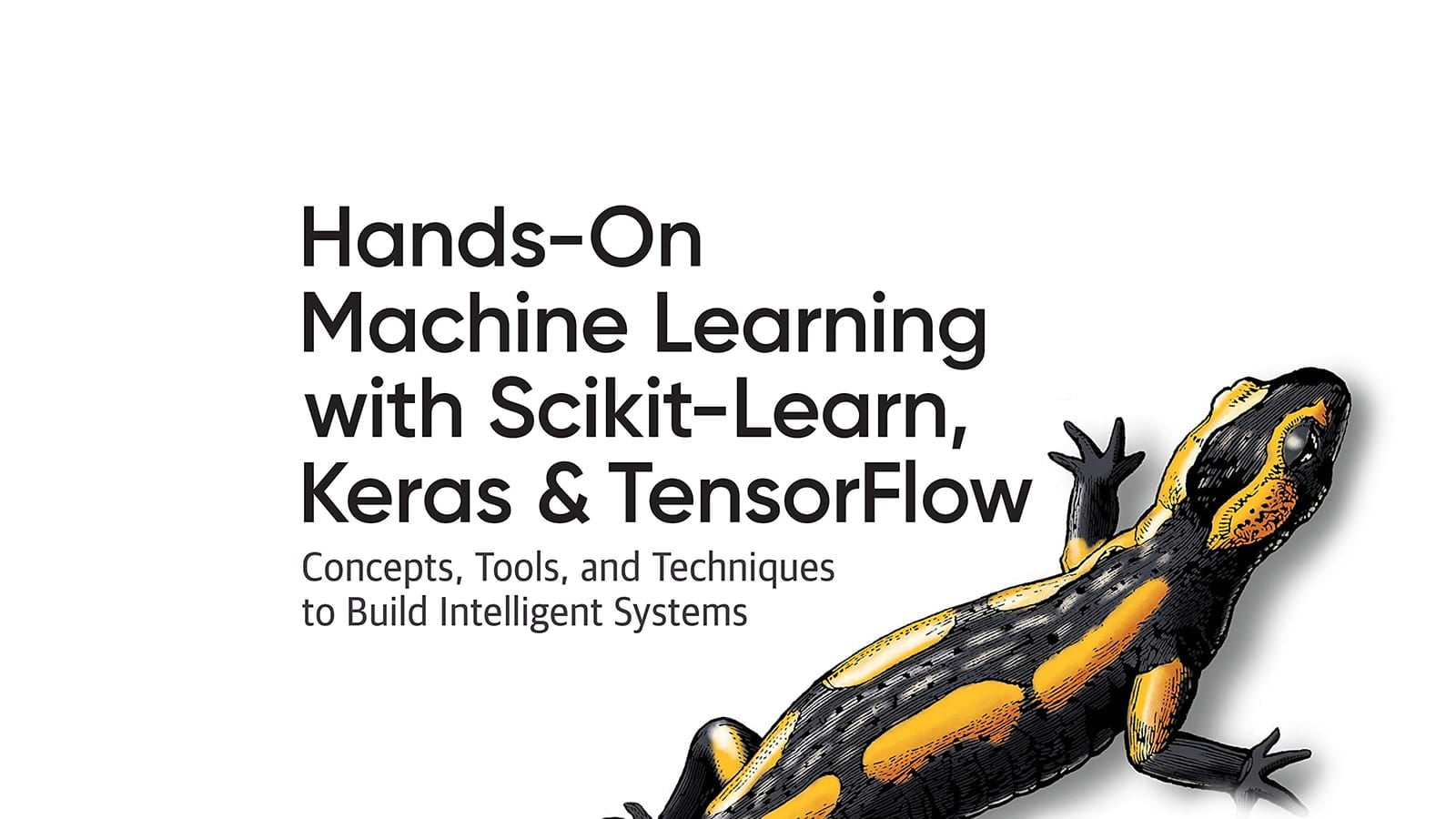
Using concrete examples, minimal theory, and two production-ready Python frameworks—Scikit-Learn and TensorFlow—this book helps you gain an intuitive understanding of the concepts and tools for building intelligent systems.
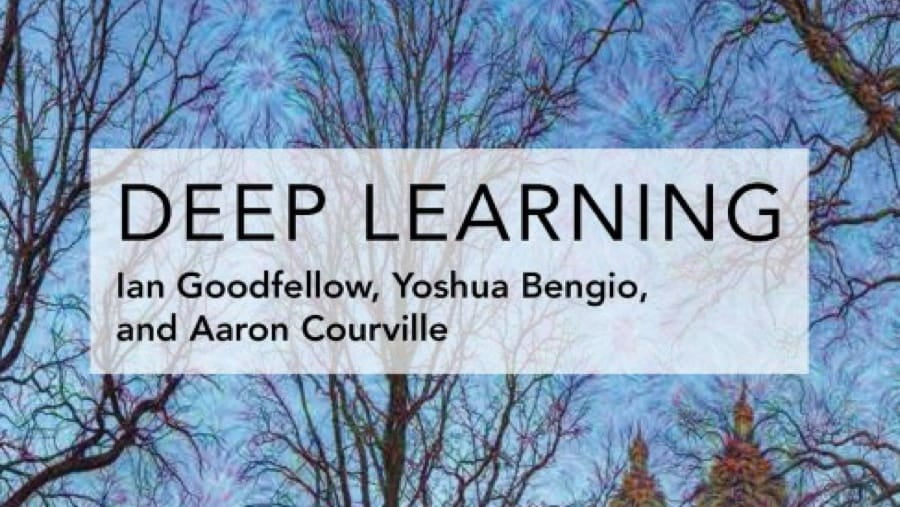
This Deep Learning textbook is a resource intended to help students and practitioners enter the field of machine learning in general, and deep learning in particular.
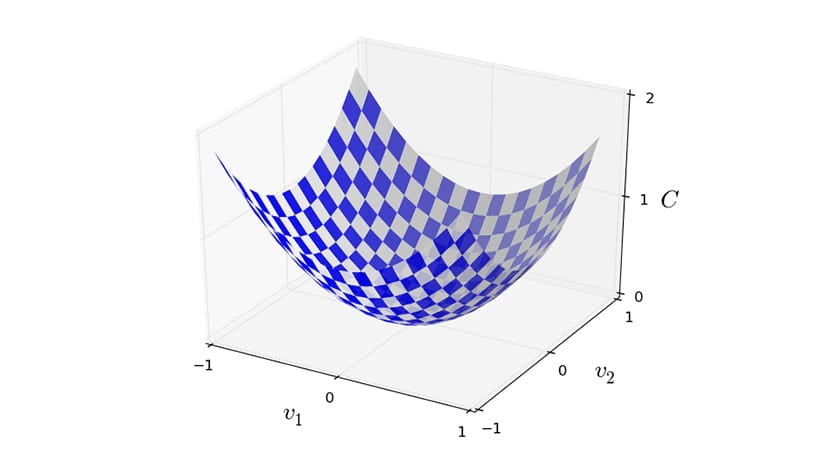
This book provides a theoretical background on neural networks. It does not use TensorFlow, but is a great reference for students interested in learning more.
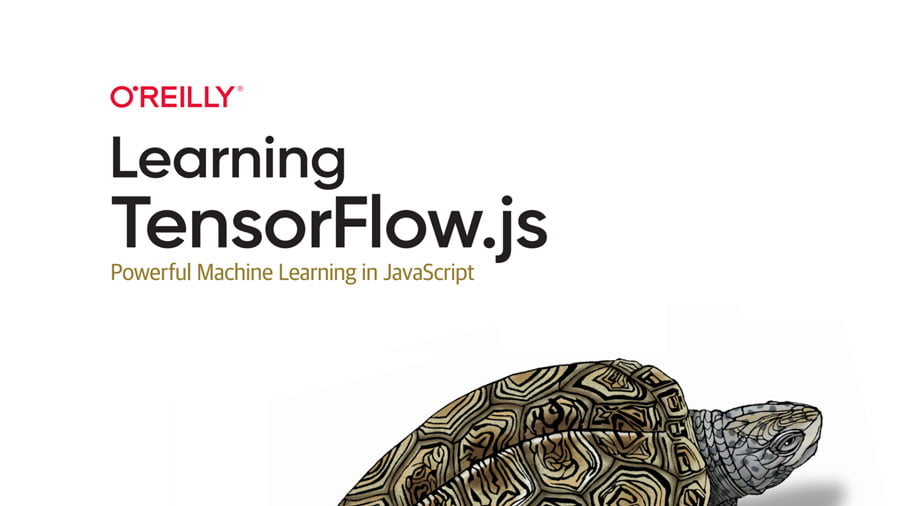
A hands-on end-to-end approach to TensorFlow.js fundamentals for a broad technical audience. Once you finish this book, you'll know how to build and deploy production-ready deep learning systems with TensorFlow.js.
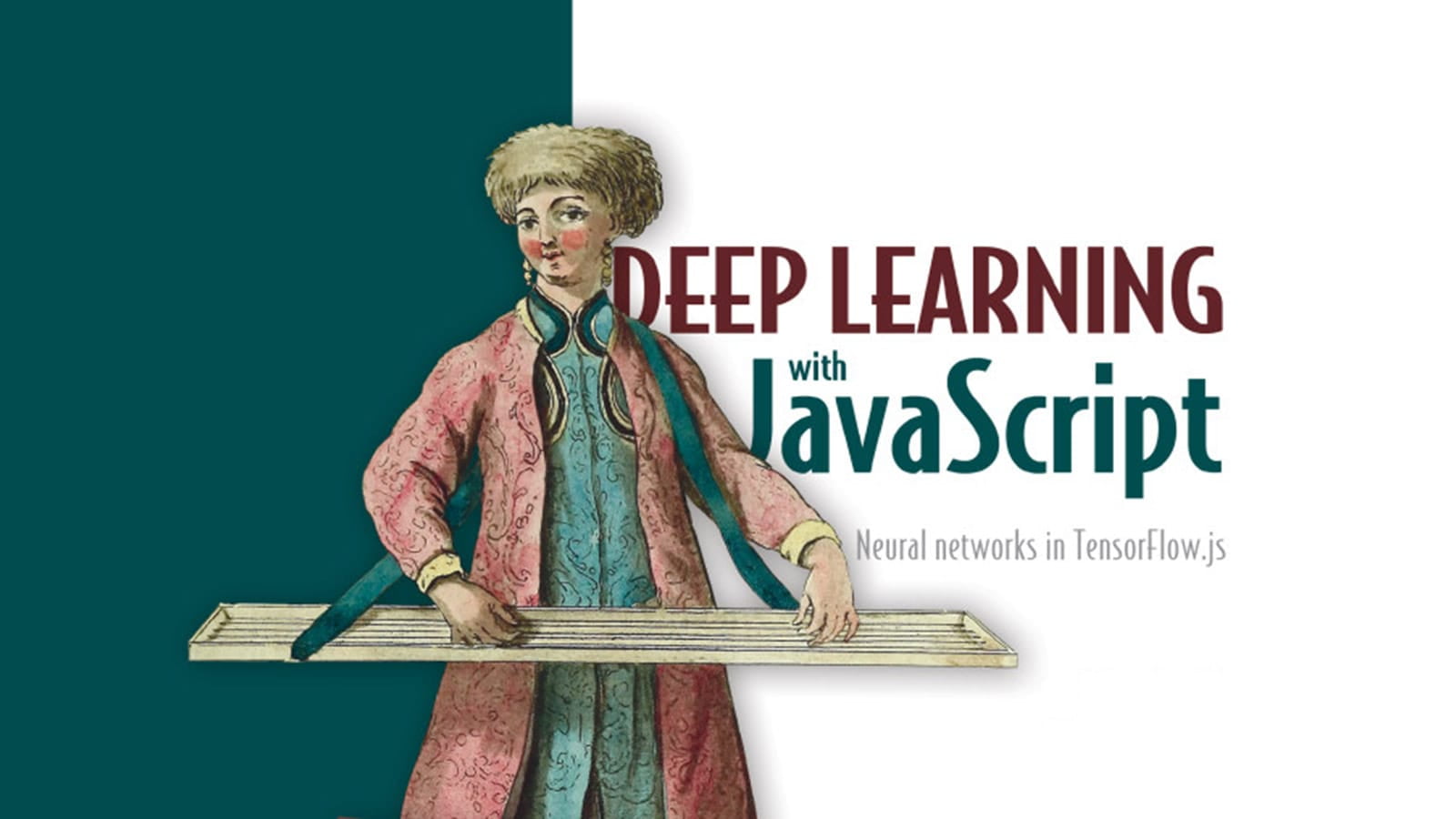
Written by the main authors of the TensorFlow library, this book provides fascinating use cases and in-depth instruction for deep learning apps in JavaScript in your browser or on Node.
Online courses
Taking a multi-part online course is a good way to learn the basic concepts of ML. Many courses provide great visual explainers, and the tools needed to start applying machine learning directly at work, or with your personal projects.
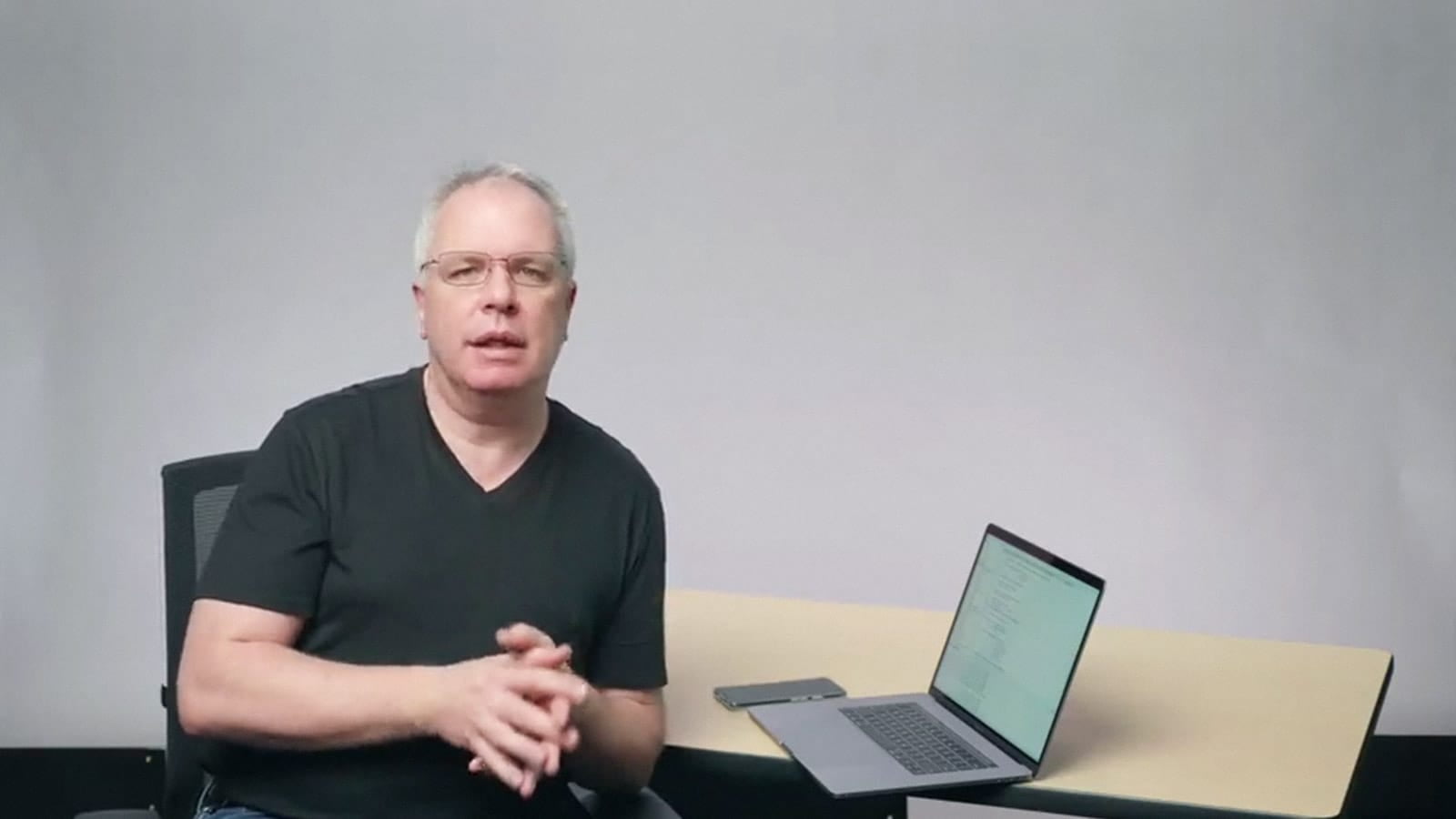
DeepLearning.AI
Intro to TensorFlow for AI, ML, and Deep LearningDeveloped in collaboration with the TensorFlow team, this course is part of the TensorFlow Developer Specialization and will teach you best practices for using TensorFlow.
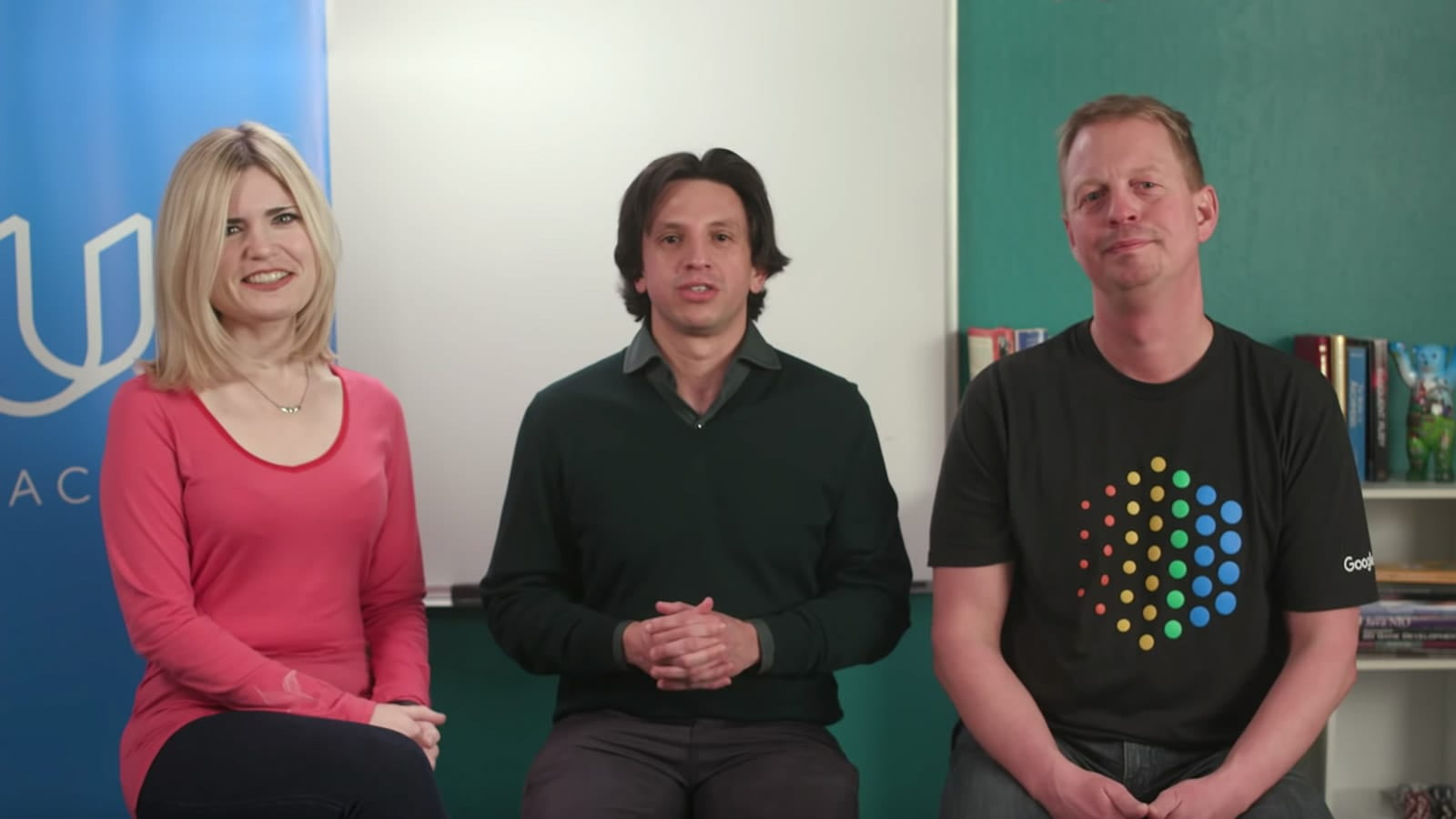
In this online course developed by the TensorFlow team and Udacity, you'll learn how to build deep learning applications with TensorFlow.
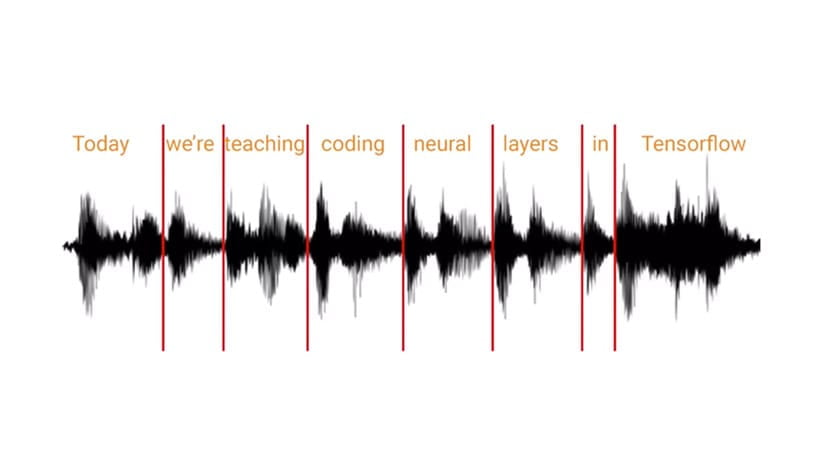
DeepLearning.AI
TensorFlow Developer SpecializationIn this four-course Specialization taught by a TensorFlow developer, you'll explore the tools and software developers use to build scalable AI-powered algorithms in TensorFlow.
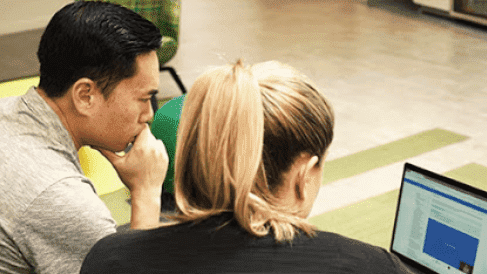
Google Developers
Machine Learning Crash CourseThe Machine Learning Crash Course with TensorFlow APIs is a self-study guide for aspiring machine learning practitioners. It features a series of lessons with video lectures, real-world case studies, and hands-on practice exercises.
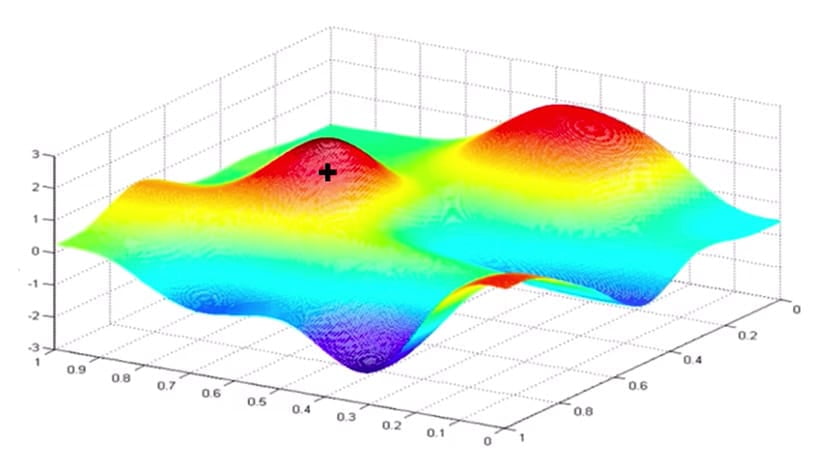
In this course from MIT, you will gain foundational knowledge of deep learning algorithms and get practical experience in building neural networks in TensorFlow.
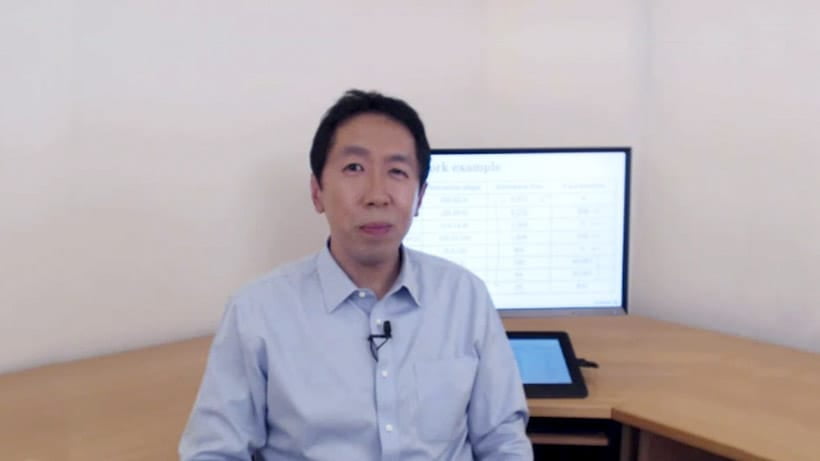
DeepLearning.AI
Deep Learning SpecializationIn five courses, you will learn the foundations of Deep Learning, understand how to build neural networks, and learn how to lead successful machine learning projects and build a career in AI. You will master not only the theory, but also see how it is applied in industry.
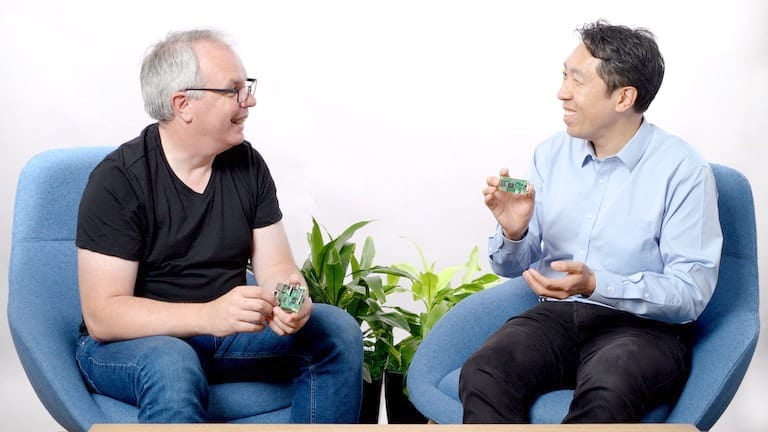
DeepLearning.AI
TensorFlow: Data and Deployment SpecializationYou've learned how to build and train models. Now learn to navigate various deployment scenarios and use data more effectively to train your model in this four-course Specialization.
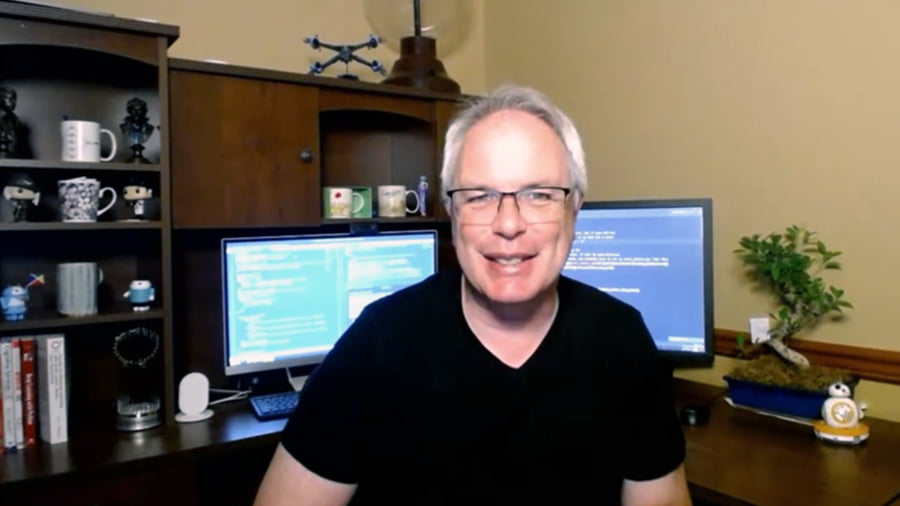
DeepLearning.AI
TensorFlow: Advanced Techniques SpecializationThis specialization is for software and ML engineers with a foundational understanding of TensorFlow who are looking to expand their knowledge and skill set by learning advanced TensorFlow features to build powerful models.
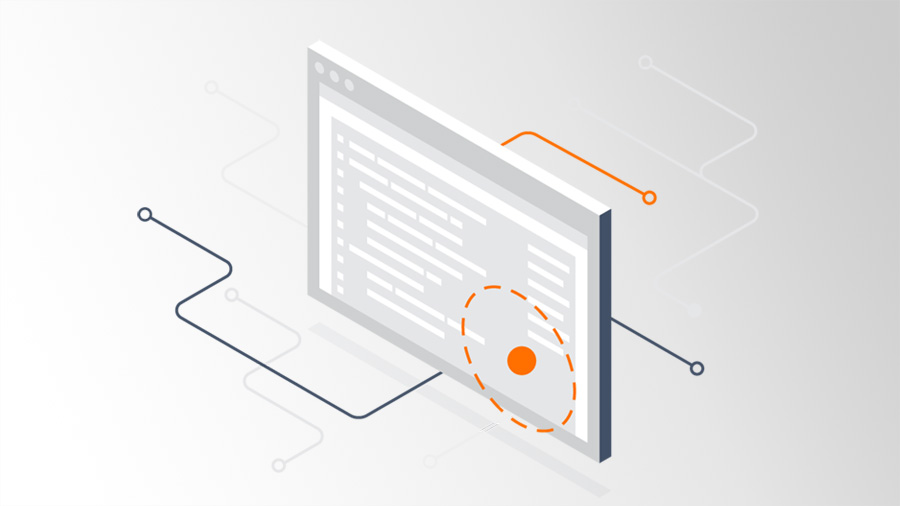
Learn how you can get more eyes on your cutting edge research, or deliver super powers in your web apps in future work for your clients or the company you work for with web-based machine learning.
Math concepts
To go deeper with your ML knowledge, these resources can help you understand the underlying math concepts necessary for higher level advancement.
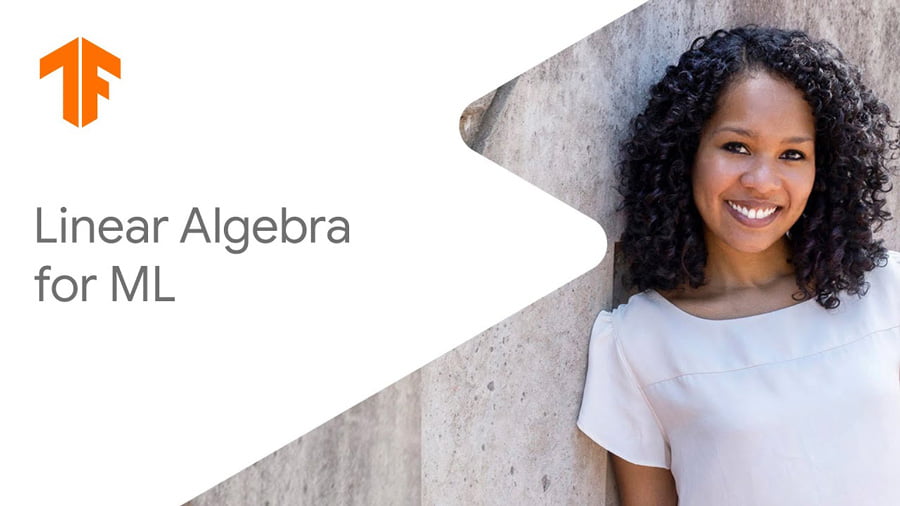
A bird's-eye view of linear algebra for machine learning. Never taken linear algebra or know a little about the basics, and want to get a feel for how it's used in ML? Then this video is for you.
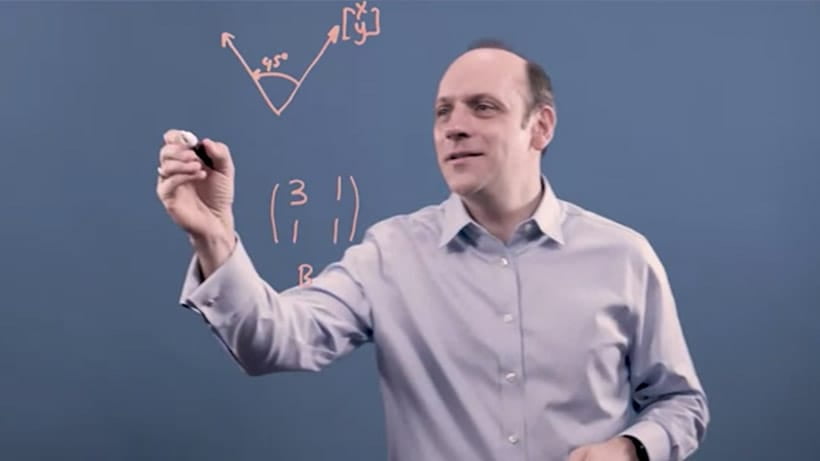
Imperial College London
Mathematics for Machine Learning SpecializationThis online specialization from Coursera aims to bridge the gap of mathematics and machine learning, getting you up to speed in the underlying mathematics to build an intuitive understanding, and relating it to Machine Learning and Data Science.
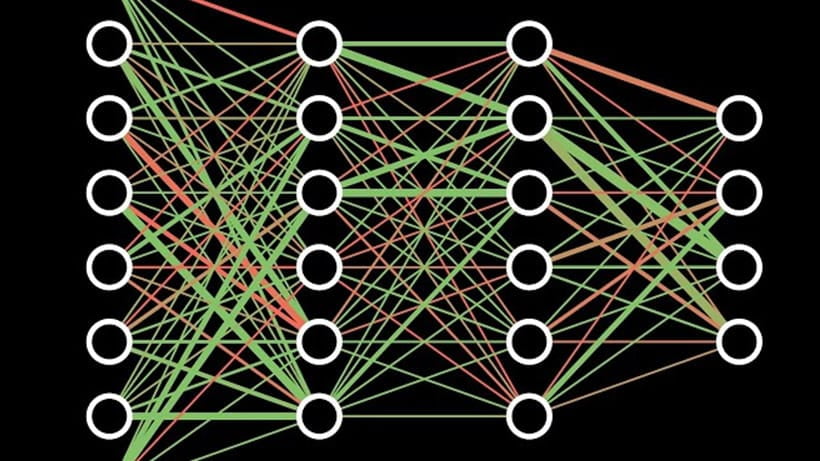
3blue1brown centers around presenting math with a visuals-first approach. In this video series, you will learn the basics of a neural network and how it works through math concepts.
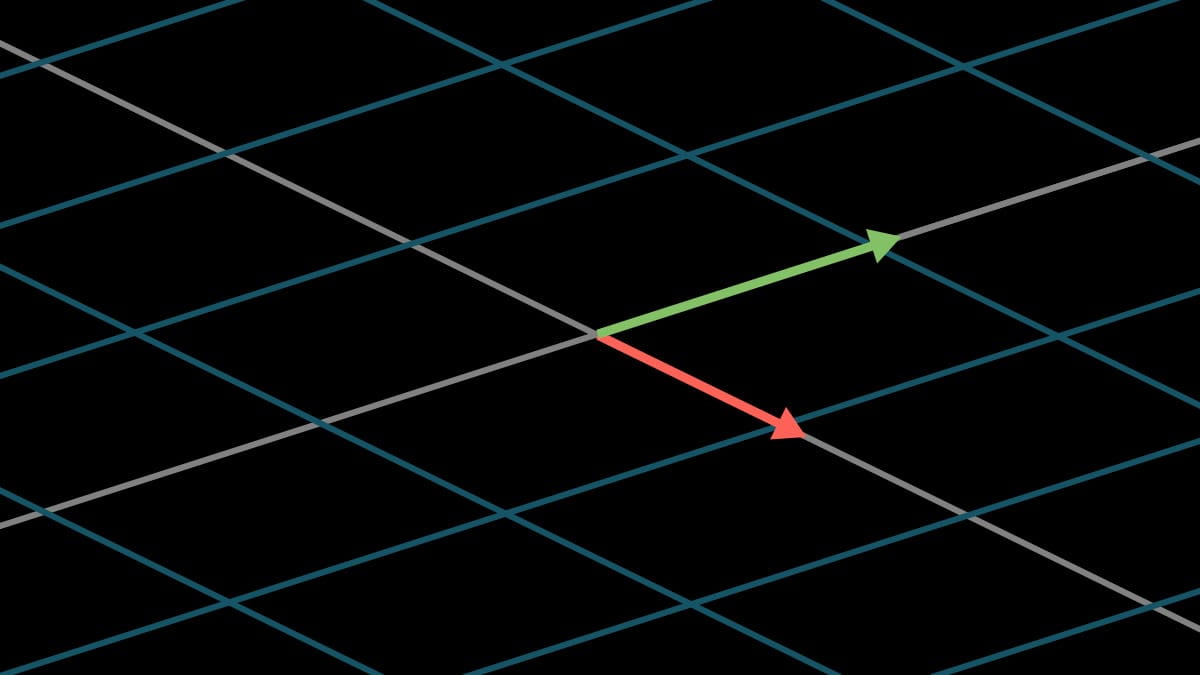
A series of short, visual videos from 3blue1brown that explain the geometric understanding of matrices, determinants, eigen-stuffs and more.
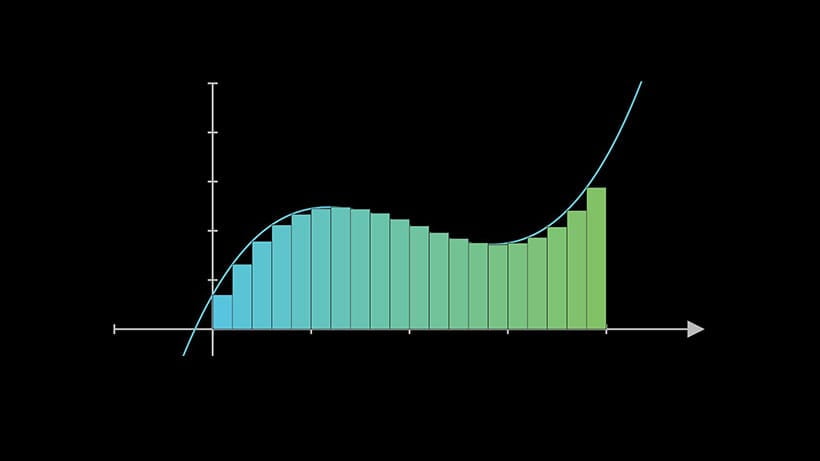
A series of short, visual videos from 3blue1brown that explain the fundamentals of calculus in a way that give you a strong understanding of the fundamental theorems, and not just how the equations work.
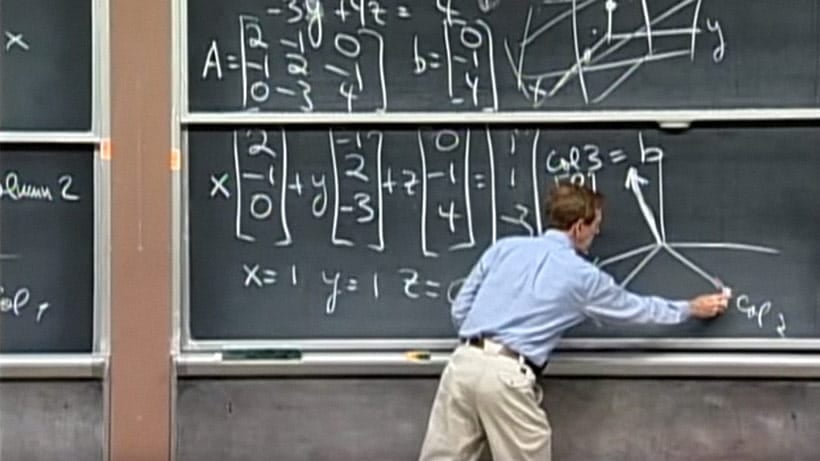
This introductory course from MIT covers matrix theory and linear algebra. Emphasis is given to topics that will be useful in other disciplines, including systems of equations, vector spaces, determinants, eigenvalues, similarity, and positive definite matrices.
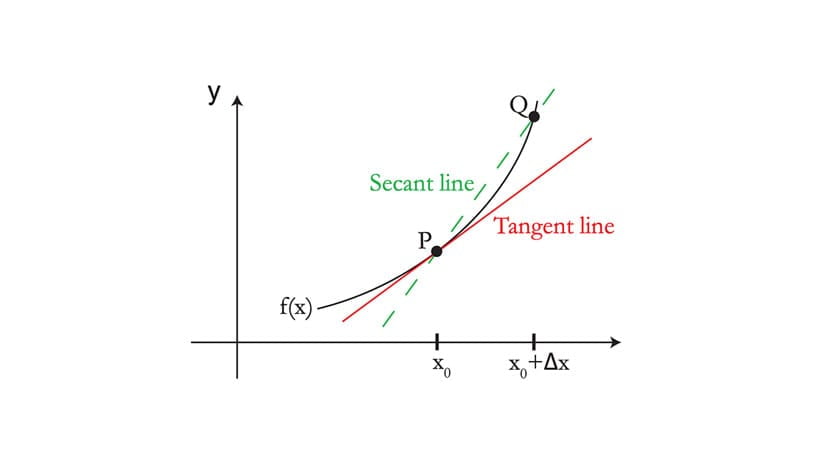
This introductory calculus course from MIT covers differentiation and integration of functions of one variable, with applications.
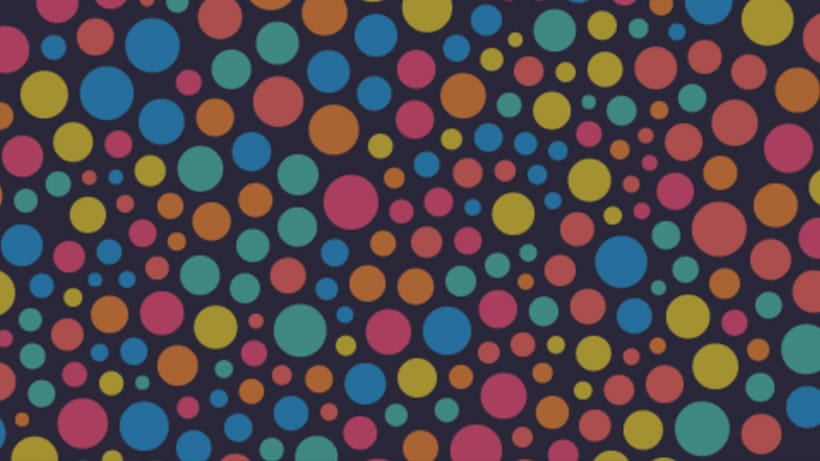
A visual introduction to probability and statistics.
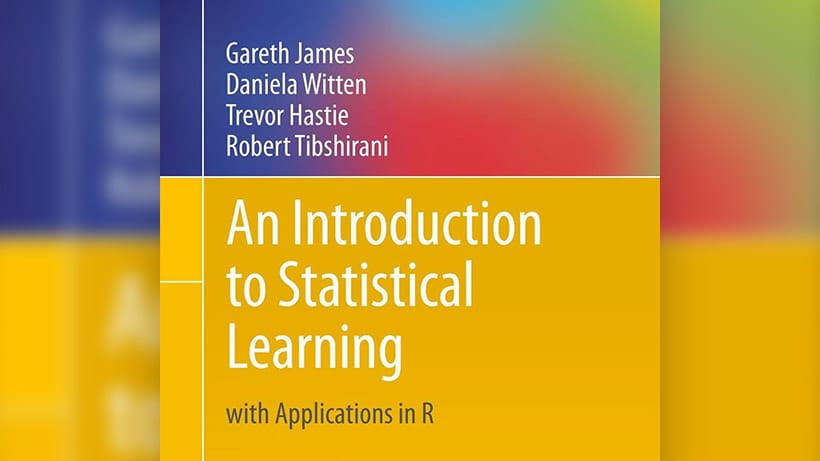
This book provides an accessible overview of the field of statistical learning, an essential toolset for making sense of the vast and complex world of datasets needed to train models in machine learning.
TensorFlow resources
We've gathered our favorite resources to help you get started with TensorFlow libraries and frameworks specific to your needs. Jump to our sections for TensorFlow.js, TensorFlow Lite, and TFX.
You can also browse the official TensorFlow guide and tutorials for the latest examples and colabs.
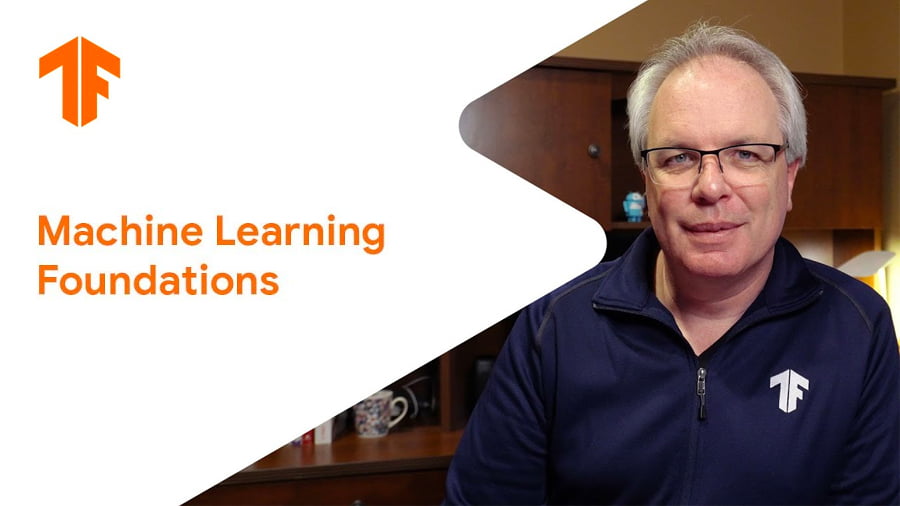
Machine Learning Foundations is a free training course where you'll learn the fundamentals of building machine learned models using TensorFlow.
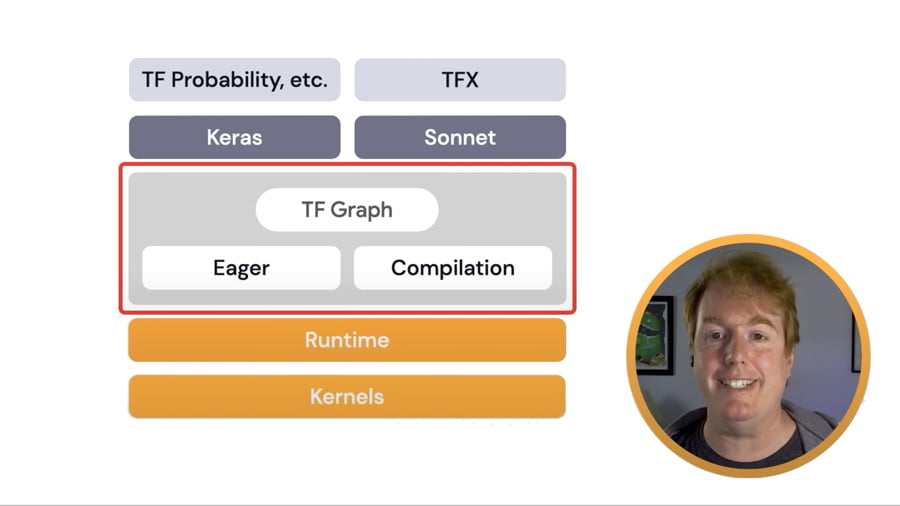
This ML Tech Talk is designed for those that know the basics of Machine Learning but need an overview on the fundamentals of TensorFlow (tensors, variables, and gradients without using high level APIs).
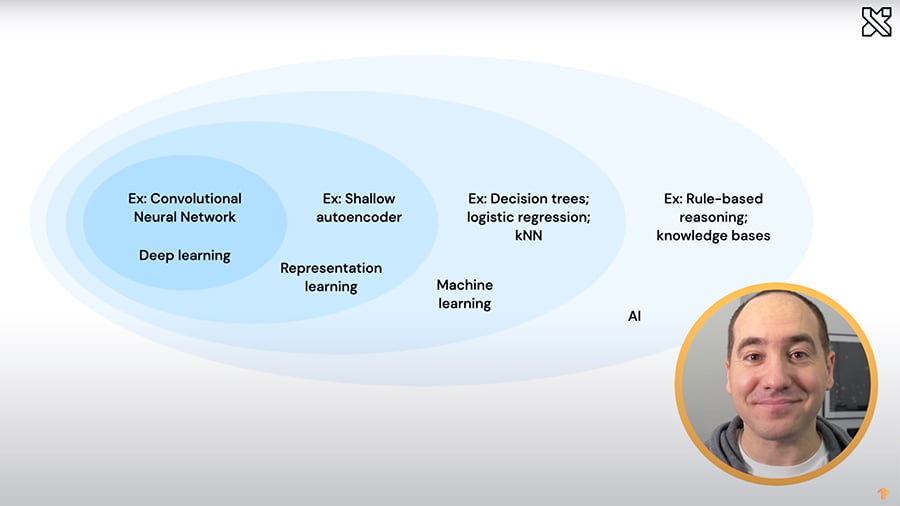
This ML Tech Talk includes representation learning, families of neural networks and their applications, a first look inside a deep neural network, and many code examples and concepts from TensorFlow.
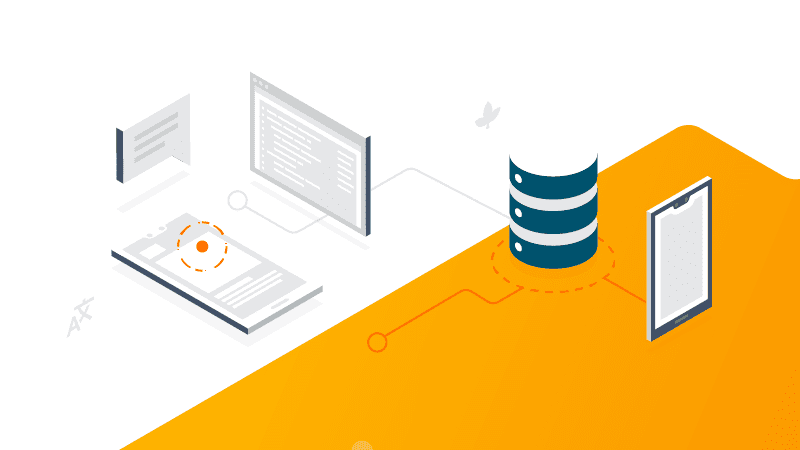
In this series, the TensorFlow Team looks at various parts of TensorFlow from a coding perspective, with videos for use of TensorFlow's high-level APIs, natural language processing, neural structured learning, and more.
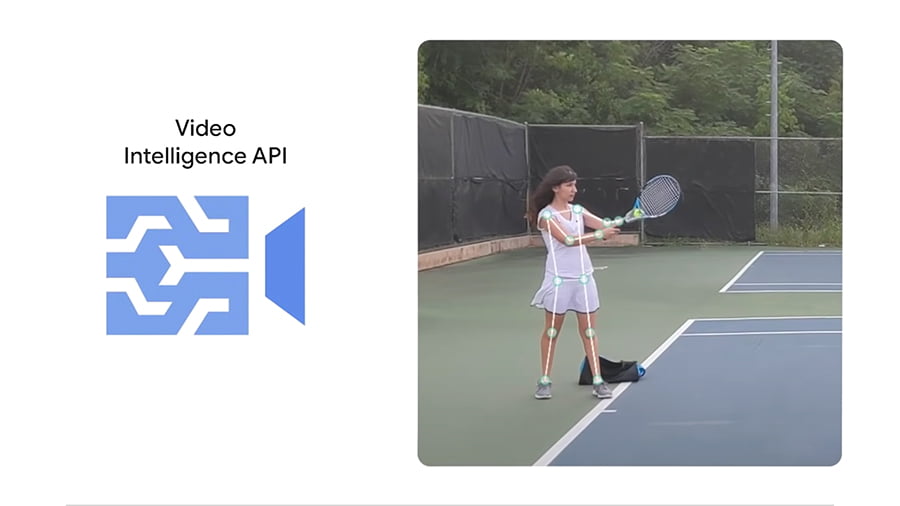
Learn to spot the most common ML use cases including analyzing multimedia, building smart search, transforming data, and how to quickly build them into your app with user-friendly tools.
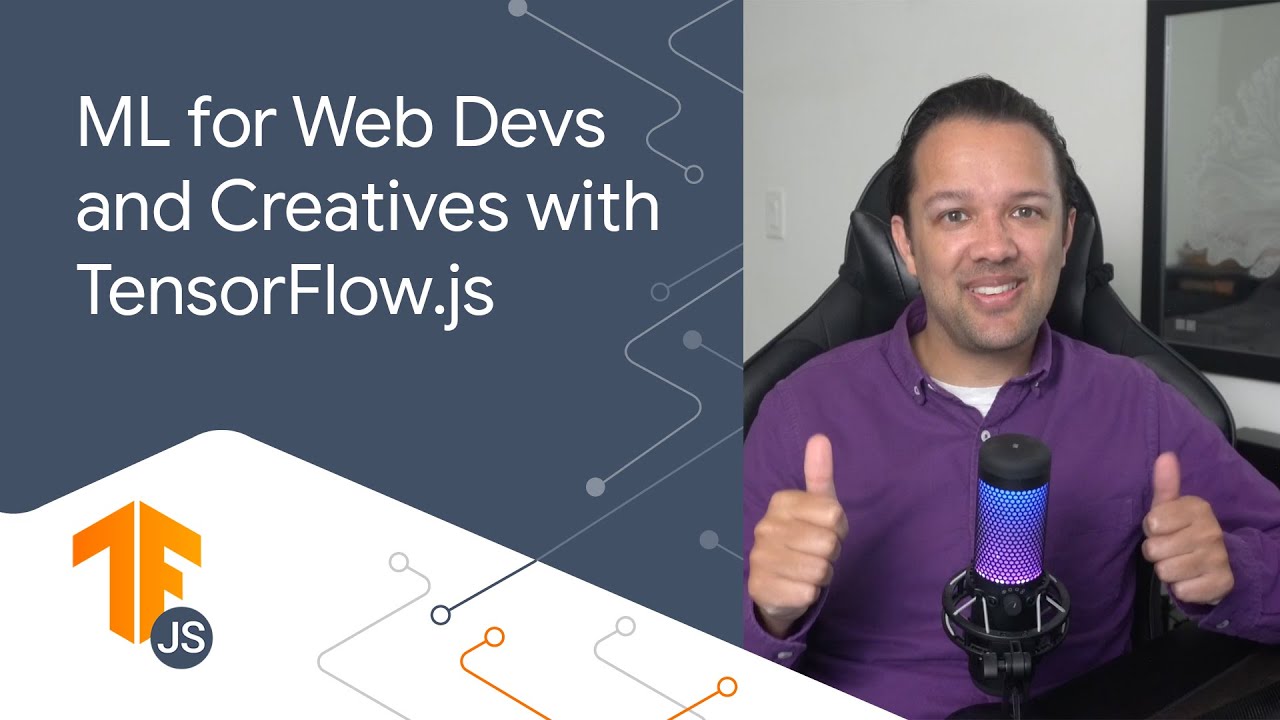
Get a practical working knowledge of using ML in the browser with JavaScript. Learn how to write custom models from a blank canvas, retrain models via transfer learning, and convert models from Python.
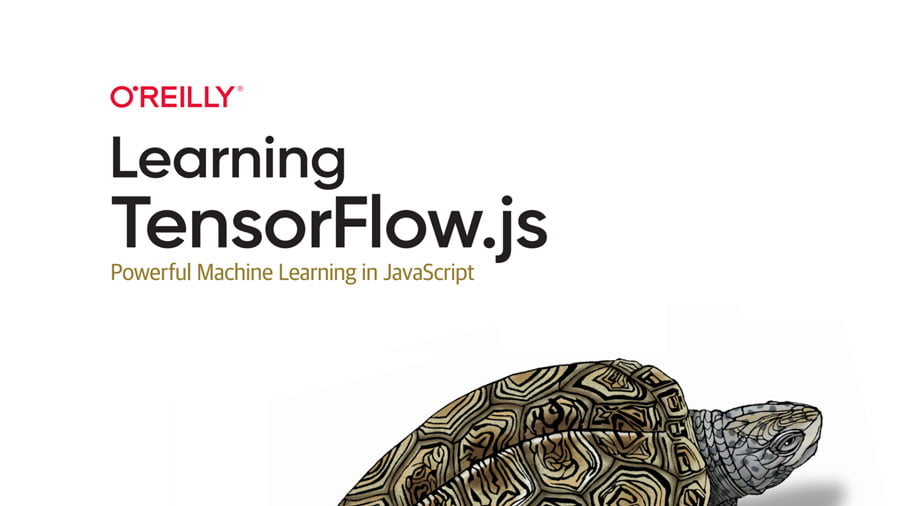
A hands-on end-to-end approach to TensorFlow.js fundamentals for a broad technical audience. Once you finish this book, you'll know how to build and deploy production-ready deep learning systems with TensorFlow.js.
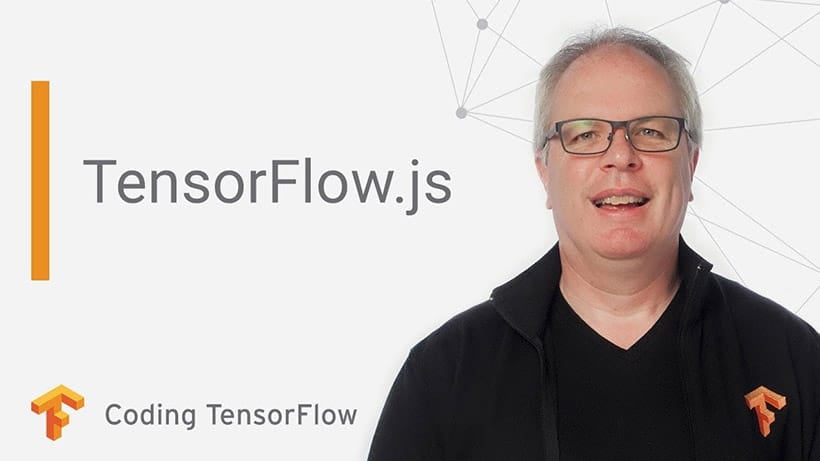
A 3-part series that explores both training and executing machine learned models with TensorFlow.js, and shows you how to create a machine learning model in JavaScript that executes directly in the browser.
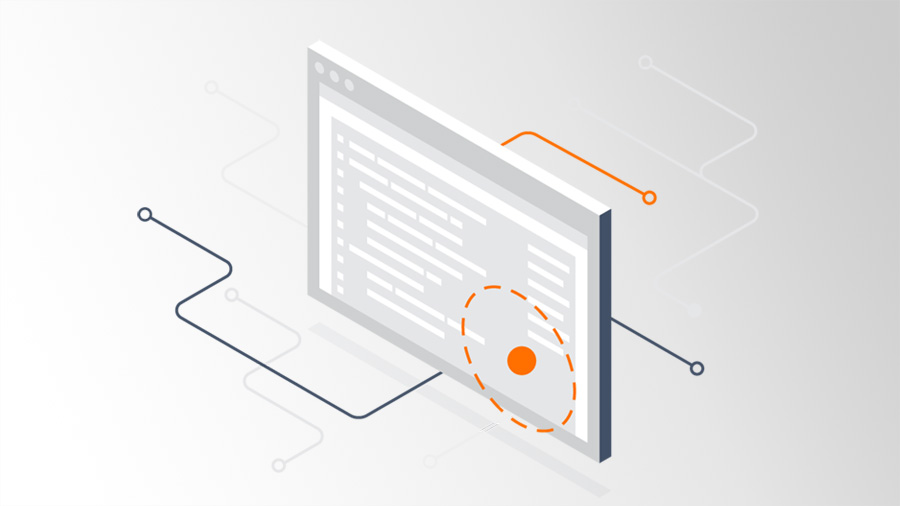
Go from zero to hero with web ML using TensorFlow.js. Learn how to create next generation web apps that can run client side and be used on almost any device.
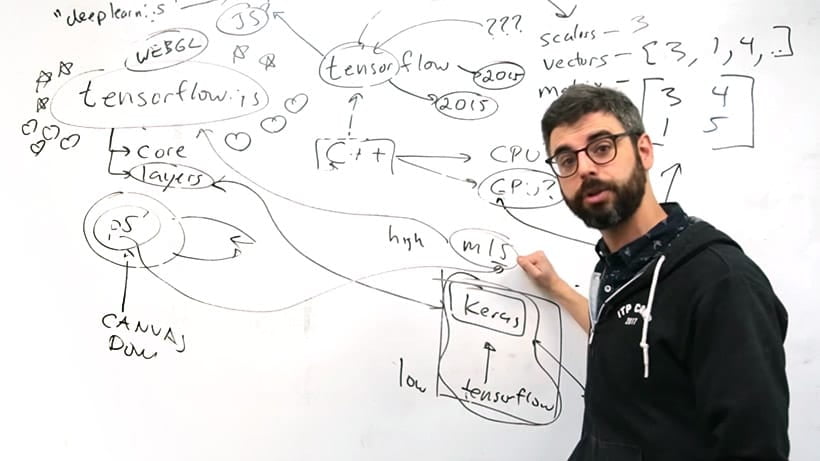
Part of a larger series on machine learning and building neural networks, this video playlist focuses on TensorFlow.js, the core API, and how to use the JavaScript library to train and deploy ML models.
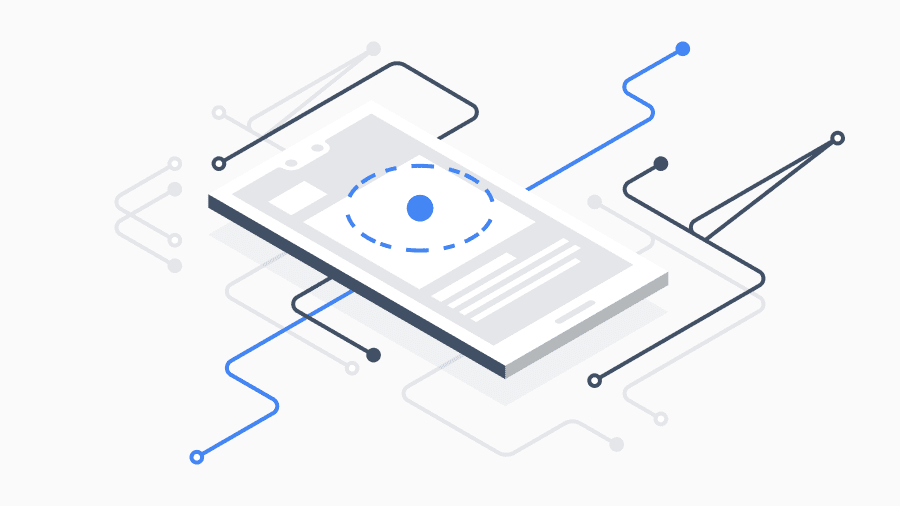
Google Developers
On-Device Machine LearningLearn how to build your first on-device ML app through learning pathways that provide step-by-step guides for common use cases including audio classification, visual product search, and more.
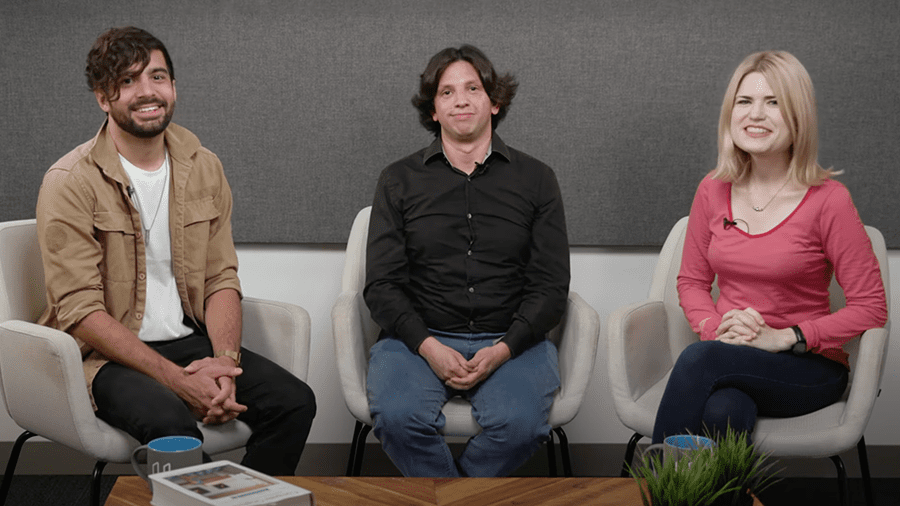
Learn how to deploy deep learning models on mobile and embedded devices with TensorFlow Lite in this course, developed by the TensorFlow team and Udacity as a practical approach to model deployment for software developers.
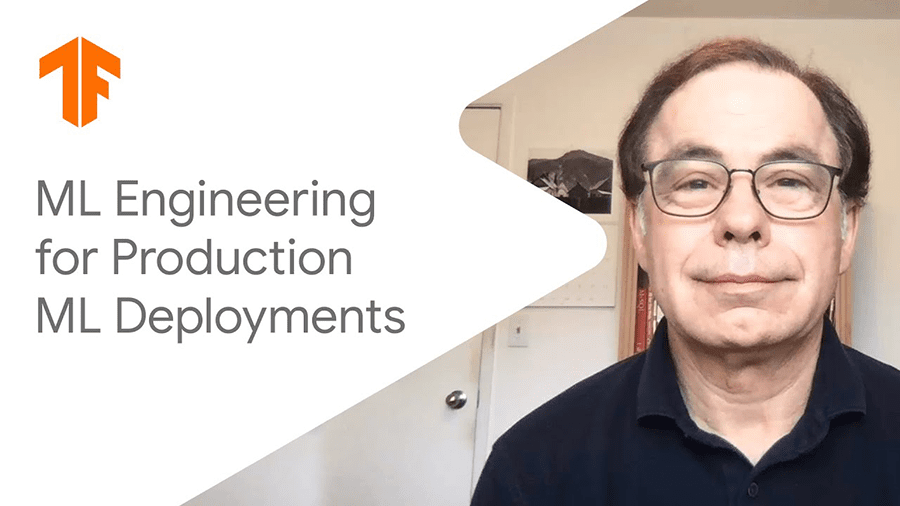
Get a hands-on look at how to put together a production pipeline system with TFX. We'll quickly cover everything from data acquisition, model building, through to deployment and management.
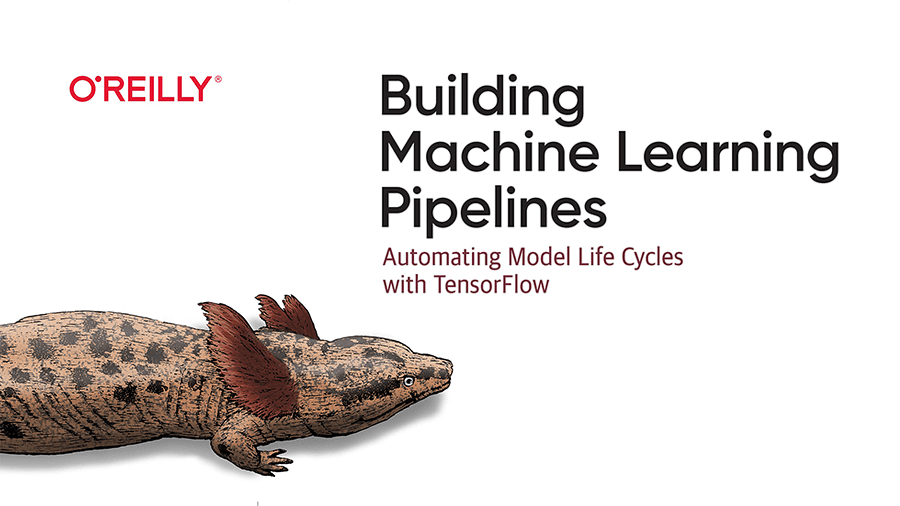
This book walks you through the steps of automating an ML pipeline using the TensorFlow ecosystem. The machine learning examples in this book are based on TensorFlow and Keras, but the core concepts can be applied to any framework.
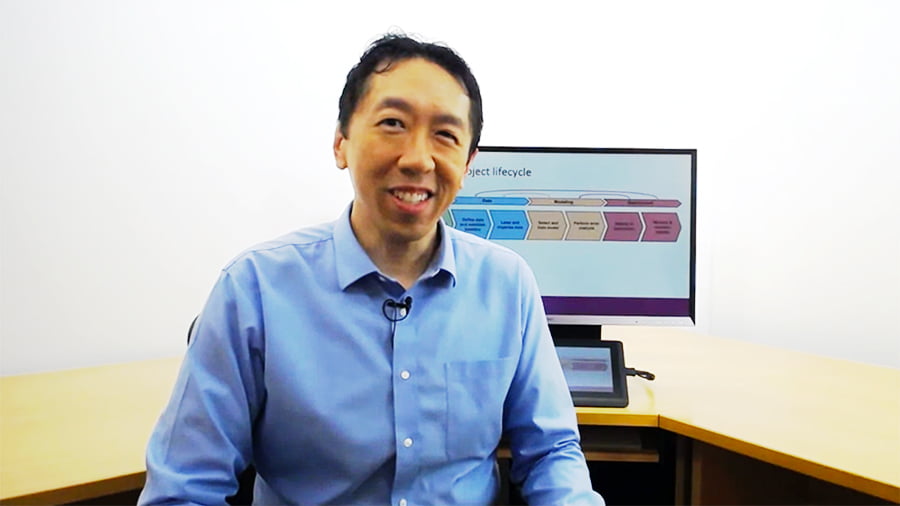
DeepLearning.AI
Machine Learning Engineering for Production (MLOps) SpecializationExpand your production engineering capabilities in this four-course specialization. Learn how to conceptualize, build, and maintain integrated systems that continuously operate in production.
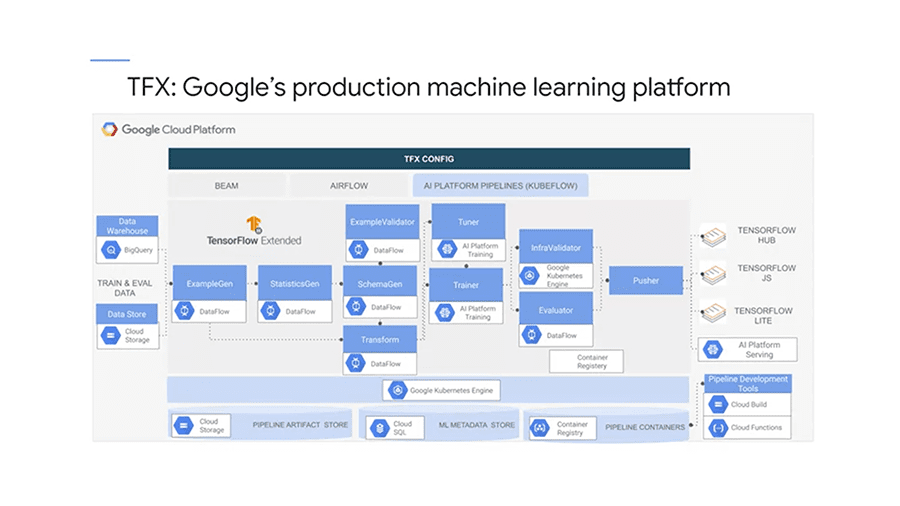
This advanced course covers TFX components, pipeline orchestration and automation, and how to manage ML metadata with Google Cloud.
Human-centered AI
When designing an ML model, or building AI-driven applications, it's important to consider the people interacting with the product, and the best way to build fairness, interpretability, privacy, and security into these AI systems.
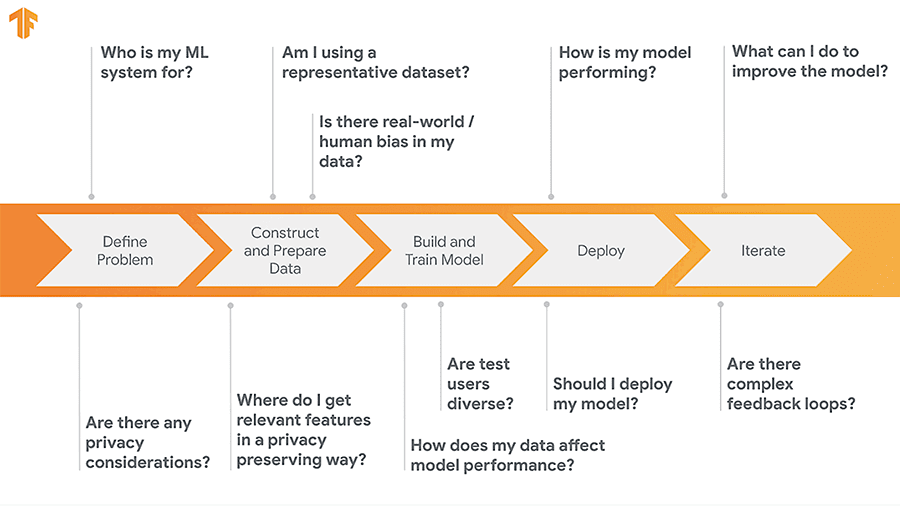
Learn how to integrate Responsible AI practices into your ML workflow using TensorFlow.
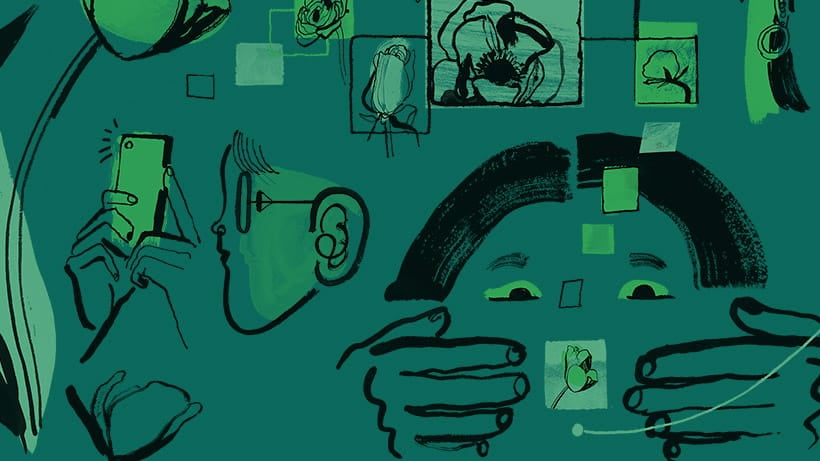
This guidebook from Google will help you build human-centered AI products. It'll enable you to avoid common mistakes, design excellent experiences, and focus on people as you build AI-driven applications.
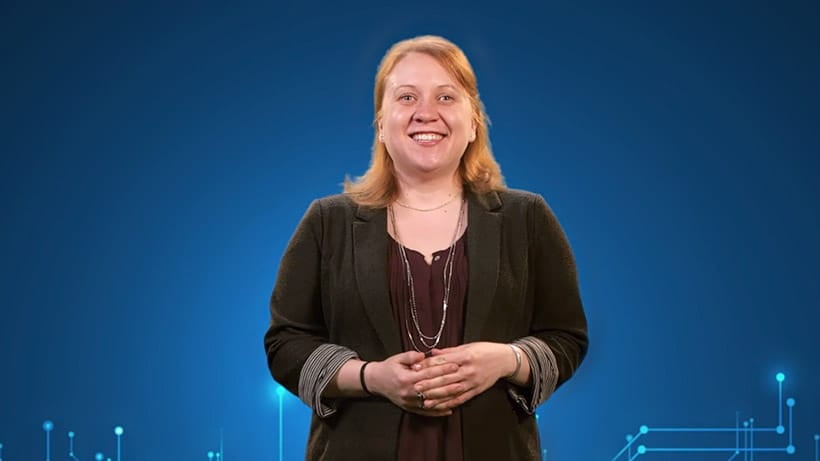
This one-hour module within Google's MLCC introduces learners to different types of human biases that can manifest in training data, as well as strategies for identifying, and evaluating their effects.